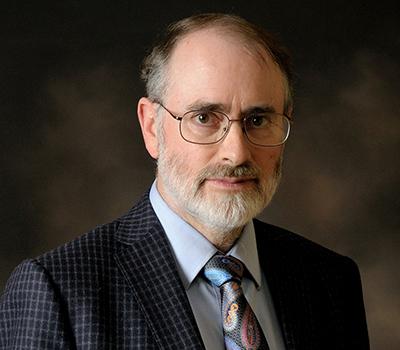
Thomas G. Dietterich
2063 Kelley Engineering Center
Corvallis, OR 97331
United States
Robust ai, machine learning, anomaly detection, computational sustainability
Thomas G. Dietterich (A.B. Oberlin College 1977; M.S. University of Illinois 1979; Ph.D. Stanford University 1984) is one of the founders of the field of machine learning. Among his research contributions was the application of error-correcting output coding to multiclass classification, the formalization of the multiple-instance problem, the MAXQ framework for hierarchical reinforcement learning, and the development of methods for integrating non-parametric regression trees into probabilistic graphical models (including conditional random fields and latent variable models). Among his writings are Chapter XIV (Learning and Inductive Inference) of the Handbook of Artificial Intelligence, the book Readings in Machine Learning (co-edited with Jude Shavlik), and his frequently-cited review articles Machine Learning Research: Four Current Directions and Ensemble Methods in Machine Learning.
Dietterich has devoted many years of service to the research community and was recently given the ACML Distinguished Contribution Award and the AAAI Distinguished Service Award. He is a former president of the Association for the Advancement of Artificial Intelligence and the founding president of the International Machine Learning Society. Other major roles include Executive Editor of the journal Machine Learning, co-founder of the Journal for Machine Learning Research, and program chair of AAAI 1990 and NIPS 2000. He currently serves on the DARPA ISAT Steering Committee and as one of the moderators for the machine learning category (cs.LG) on arXiv.
Related Podcasts
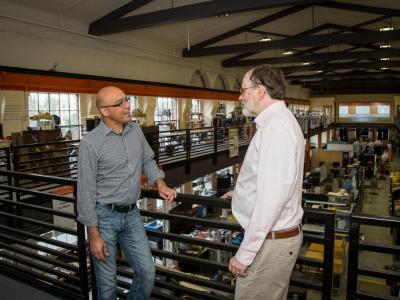
