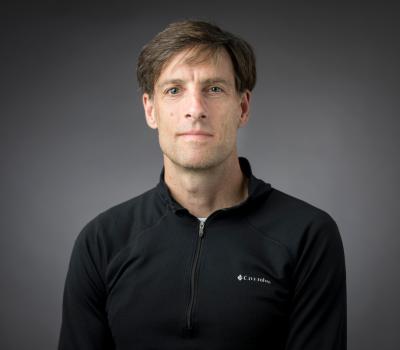
Alan Fern
GRAF 326
Corvallis, OR 97331
United States
Reinforcement learning, planning, humanoid robotics, agricultural AI
Alan Fern is a professor of computer science, artificial intelligence, and robotics in the School of Electrical Engineering and Computer Science at Oregon State University. He received his Ph.D. (2004) in computer engineering from Purdue University, and his B.S. (1997) in electrical engineering from the University of Maine. His research interests span a variety of topics in artificial intelligence and robotics with a particular emphasis on building systems that can learn from experience. He co-directs the Dynamic Robotics Laboratory with Jonathan Hurst at Oregon State where he works on AI for enabling humanoid robots to do real-world work. He is also the AI director for the AgAID National AI Institute in collaboration with Washington State University, which has the mission of developing AI and robotics solutions for important problems in specialty crop agriculture.
Related Podcasts
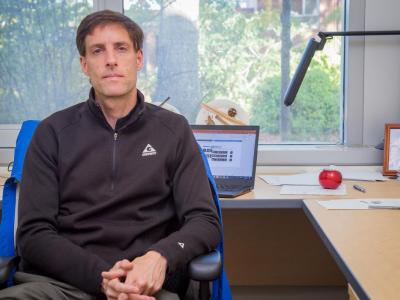
