Image
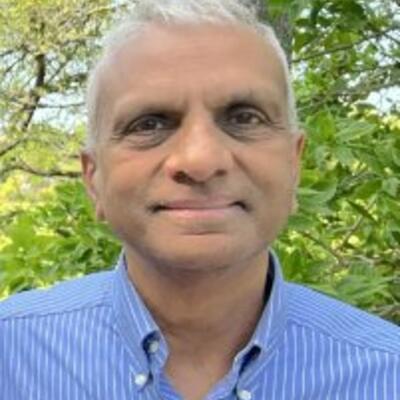
Event Speaker
Balas Natarajan Kausik
Event Type
Artificial Intelligence
Date
Event Location
KEC 1001 and Zoom
Event Link
Event Description
Gradient descent on large neural networks such as transformers overfits early with many small weights, and therefore inefficient on both computing resources and training data. In response to these inefficiencies, we exploit learning theory to derive Occam Gradient Descent, which interleaves optimal reduction of model size to reduce test loss, with gradient descent on model weights to reduce training loss. Our experiments show that with respect to loss, compute and model size, Occam Gradient Descent outperforms traditional gradient descent across a range of problem domains.
Speaker Biography
B.N. “Nat” Kausik is an independent researcher and entrepreneur. A graduate of Cornell, Princeton and IIT Madras, he has worked in both academia and industry.
Video Recording