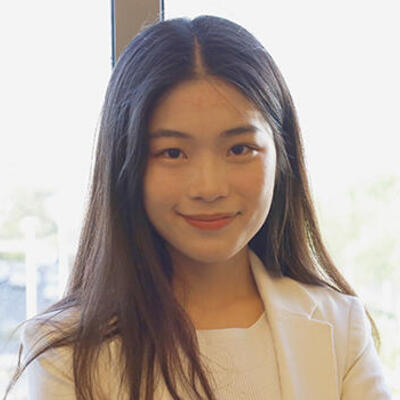
Large-scale scientific simulations drive scientific and engineering discovery across many domains, but face performance problems. These simulations typically involve complex physics computations but are difficult to scale efficiently on high-performance hardware. Machine Learning (ML)-based surrogates are revolutionizing the natural sciences. By employing reverse-engineering and automatic learning methodologies, ML surrogates can solve complex, unstructured problems with less computing power and execution time than needed by traditional direct and first-principle methods, with the advantages of lower implementation costs, stronger generalization capability, and lower computation overheads. In this talk, I will introduce the application of ML-based surrogates in two user-inspired High-Performance Computing (HPC) applications, and a generalized framework that automates the application process of ML-based surrogates in the HPC domain. Auto-HPCnet democratizes the usage of ML-based surrogates and is the first end-to-end framework that makes past proposals for the ML-based surrogate model practical and disciplined. Auto-HPCnet introduces a workflow to address unique challenges when applying the surrogates, such as feature acquisition and meeting the application-specific constraint on the quality of the final computation outcome. Also, the two real-world HPC applications include the Eulerian fluid simulation and the AC-OPF power grid simulation. In the Eulerian fluid simulation, we generate multiple surrogate candidates before the simulation and introduce a runtime scheduler that dynamically switches the ML surrogates to make the best efforts to reach the user’s requirement on simulation quality. We show that our method achieves 1.46x and 590x speedup, compared with a state-of-the-art ML model and the numerical fluid simulation respectively, while providing better simulation quality than the state-of-the-art model. In the AC-OPF power grid simulation, we generate multitask-learning (MTL) surrogates to predict the initial values of variables critical to the convergence of the power grid problem. The MTL models allow information sharing when predicting multiple dependent variables, while including customized layers to predict individual variables. The MTL model incorporates physics-informed learning to improve model accuracy and interpretability. These techniques bring 2.60× speedup on average (up to 3.28×) computed over 10,000 large-scale power grid problems, without losing solution optimality.
Wenqian Dong is an assistant professor in the School of Electrical Engineering and Computer Science at Oregon State University. She earned her Ph.D. in EECS at the University of California, Merced, in Spring 2022. Recently, she is selected for the IEEE-CS Technical Community on High Performance Computing (TCHPC) Early Career Researchers Award for Excellence in High Performance Computing. Her research focuses on three main areas. She has contributed significantly to scientific machine learning, particularly in using machine learning to speed up HPC applications. Her work is showcased in conferences like SC’19 and SC’20. Wenqian has excelled in automatic machine learning, concentrating on creating machine learning models for HPC applications. Her papers in VLDB’21, HPDC’23, and ASPLOS’22 highlight her notable contributions. She’s skilled in optimizing system performance, aiming to enhance HPC applications’ quality and efficiency through system optimization. Her work presented at conferences like ICS’21, Eurosys’21, ICPP’18, and Parallel Computing’23 illustrates her dedication to this field. Her work has generated real impacts in the HPC community. For example, her work on power grid simulation using ML leads to 3.28 times performance improvement and highlighted Newswise as a DOE science innovation. Furthermore, Wenqian is committed to enrich the HPC community. Her commitment is apparent in her various roles as an organizer for ICPP’24, the MLBench’23 workshop, and as a member of the Technical Program Committee (TPC) for the SC’24, HPDC’24, CCGrid’24, IEEE Cloud 2023, IEEE Cluster 2023, AI4Science 2022 workshop, and the GPGPU 2023 workshop.